Helping Students Stay the Course: 5 ways predictive analytics can advise when to offer support
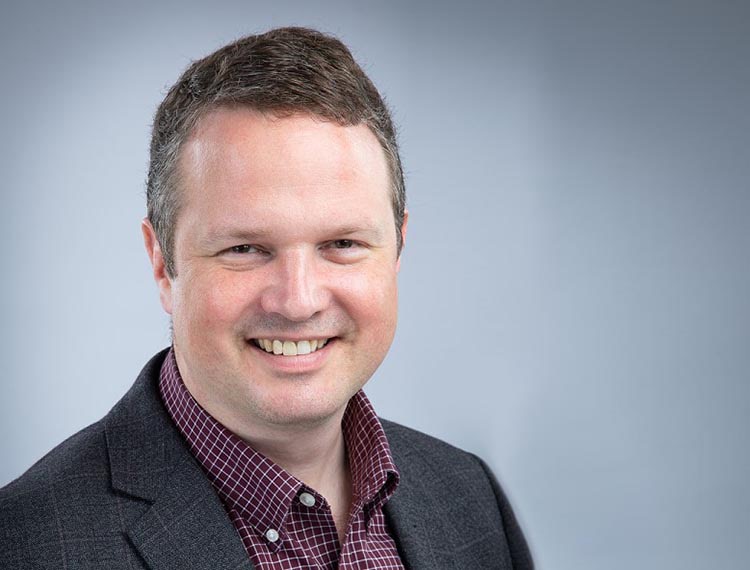
Artificial Intelligence in Education: Using #Predictive Modelling, #MachineLearning and #AI to boost Student Retention
In August, 364,380 A-Level students won places at British universities, including 33,630 overseas students.
However, a growing percentage of undergraduates leave before completing their degrees.
Latest figures from the Higher Education Statistics Agency (HESA) from 2016/2017 show that 10.4 per cent of UK undergraduates neither gained an award nor transferred to other courses.
Dropping out of a university course can have a heavy impact on students’ emotional wellbeing and financial outlook, as well as impacting the wider economy through loss of skills.
A number of universities in the US have turned to predictive analytics to help identify students who are at risk of transferring or dropping out completely, so that they can be offered counselling, additional tutorial time, and financial support.
1. Tailoring admissions
Often, student retention can be improved at the outset, by matching candidates more effectively to universities and courses.
The admissions department at Taylor University in Indiana has successfully improved its student retention by using analysis of historical data from College Board, ACT, NRCCUA, public census data and financial aid data, to identify students who are most likely to enrol.
Using the combined data sets, the university built a predictive analytics model in WebFOCUS RStat. The resulting admissions InfoApp compares current prospective students to past students, to help university staff to observe patterns.
Analysis of this combined data set is then used to help 5 recruiters to whittle down 50,000 prospective students to 2,000 people who share the characteristics of successful alumni. The university’s marketing team then conduct targeted recruitment campaigns to these potential applicants, from which 500 students will be offered places.
2. Enriching campus life
Once they enrol, Taylor University’s Faculty and Staff department uses the same analytics platform to more precisely tailor extra-curricular events to students’ needs. Schedule TU is an information app that merges academic calendars to identify the availability of teaching spaces and people on campus. As an example, Schedule TU allows sports coaches to identify when student athletes are available for practice, without disrupting their lectures.
As they progress through their studies, Taylor University also applies predictive analytics to identify students who might be struggling financially, academically, or socially. If students are finding their courses too challenging, the Faculty and Staff department can refer them for mentoring at the university’s Academic Enrichment centre.
“With the right guidance, we can work with a student to prevent them from dropping out or transferring,” comments Edwin Welch, Director of Institutional Research at Taylor University.
3. Matching ingredients for success
Taylor University has applied analysis of historical data, predictive modelling, machine learning and data mining to better match students to courses and extra-curricular activities and to identify those at risk of dropping out.
The head of the university’s IT Enterprise Data Systems team, Mark Lora, explains:
“We identified the set of conditions that equate to success at each phase of the lifecycle and then looked for patterns indicating that a student is heading in that direction. The machine-learning algorithms in RStat identify the variables that help us predict student achievement through the recruitment cycle.”
4. Improving financial support
According to HESA figures, students from less wealthy families are at higher risk of dropping out of college.
Michigan’s Davenport University, used analytics to help reduce the time taken to calculate student financial aid by 80% and has improved student retention by two percentage points every year for 5 years running.
The student retention report also uses analytics to identify students who might need academic intervention, such as one to one tutoring, “By identifying students who are close to completing and encouraging them to complete degrees – via counselling or extra financial aid – this persistence metric is steadily rising,” says Marylee Van der Heuvel, Senior Data Warehouse Administrator at Davenport University.
The use of analytics also helps to improve efficiency for the university and students. If a student leaves midway through a term, the university has to return the unused portion of any financial aid to the government. In order to calculate the sum that needs to be repaid, various factors have to be taken into account including the number of tutorial days completed, minus holidays and sick days; the charges for that student’s course; and the mix of grants to loans.
Davenport University’s executive director of financial aid, David DeBoer explains, “We use WebFOCUS to gather data from about two dozen tables, perform the calculations, and load the results into designated fields in an Excel spreadsheet.”
The analytics platform automates the calculations to save the university from having to manually calculate financial awards and formats the information in a way that is easy to review and audit. The same platform notifies the financial aid team if a student changes course, such as moving from an associate degree to a bachelor’s degree, which affects their financial aid package.
DeBoer estimates that analytics has reduced the time taken to calculate financial packages from half an hour per student to under five minutes. “It saves us hundreds of hours each year and my team no longer has to generate 10 to 15 pages of documentation for each student.”
5. Offering emotional support
Davenport University has also explored using predictive analytics to identify at-risk students so that they can be invited in for counselling. Counsellors can also compare the progress of current students with previous student data, to tailor and improve the support offered.
Similarly, Central Washington University has used WebFOCUS RStat to create a risk analysis model to predict which students might drop out. Reports are automatically generated for college counsellors, so that students can be invited to come in for advice and support. “We have a good national ranking in these areas but we want to do better,” asserts James DePaepe, executive director for Accreditation, Comprehensive Planning, and Organizational Effectiveness.
DePaepe’s team created the risk analysis model using the same data analytics platform that helped streamline mandatory reporting to the Public Centralized Higher Education Enrollment System (PCHEES); US News and World Report, Petersons and ACT. Prior to implementing the platform, the team had to create more than 100 internal reports each year, with the PCHEES report alone taking around 8 months to complete.
Central Washington University’s investment the platform has enabled staff to use self-service analytic reports, which has freed up the Accreditation, Comprehensive Planning and Organizational Effectiveness team to focus on analysis of admissions, student retention figures and graduation rates.
“As our reporting requirements escalated, there was an acute demand for an analytics and reporting system that could provide a historical perspective, allow for trend analysis, as well as support custom self-service analytical and dashboard reporting,” notes DePaepe.
To marry its federal reporting requirements and student welfare monitoring Colorado State University has invested in a hybrid on-premise data and cloud-based analytics platform which allows college administrators to monitor student retention, attrition and graduation rates. Colorado State University has used the platform to develop its Student Success InfoApp™ and Degrees InfoApp which assist the staff in analysing degrees awarded by gender, ethnicity, degree category, and other variables, so that resources can be allocated appropriately to support future students’ success.
While three quarters of a million students look forward to freshers’ weeks up and down the country, HESA figures indicate that almost one in ten will not return for their second year.
Recent research “Artificial Intelligence in Education” has investigated how students’ behavioral signals and incoming profiles can be integrated to describe and predict student success in a higher education’s STEM course. The results include three major findings:
These findings can set the stage for subsequent early warning system studies that use different types of student data. |
Using analytics to rapidly review decades of data relating to student retention and successful graduation rates, universities can identify the patterns that indicate which factors affect student welfare and enjoyment of courses and campus life.
By using historical analysis and predictive analytic models and information apps that are easily understood by faculty staff and administrators, universities can help match students to the right places and courses at the outset and offer support at the right time to encourage students to stay the course.
Jake Freivald, Vice President of Product Marketing, Information Builders
Responses