Not all Labour Market Information is born equal
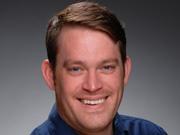
The phrase Labour Market Information (LMI) is perhaps not the sort of phrase that is likely to get people excited. In fact, I can well imagine that in the ears of many it probably sounds rather dull. For me, however, I am hugely excited about the possibilities that LMI has to really make big changes to colleges, to local economies and above all to the lives of individual people.
Does that sound over the top? I don’t believe it is, and I have seen numerous examples of colleges that have used LMI to make massive differences to the people they serve: LMI used to improve curriculum planning; LMI used for better employer engagement; LMI used to give direction and vision to students and prospective students; LMI used to measure the great value that colleges bring to the local economy; and even LMI used to make Ofsted happy – ultimately LMI can be used as a primer to bridge the gap between student perceptions and labour market realities. These are things you all care about, and so even though the phrase “Labour Market Information” might not necessarily float your boat, the applications and opportunities that LMI can bring to your college, your local economy, and to your students probably do.
My passion for LMI then is not so much a passion for data, but a desire to see colleges use data in order to become what Suzanne Duncan described here as “economic dynamos in the heart of their community”. What does she mean by that?
For LMI to really help you, it obviously needs to give you an accurate picture of what is going on in your local labour market. This is not actually as simple as it sounds. The raw data that comes from government sources is actually full of “holes”, and it is notoriously difficult to find out what is actually going on in those “holes” – that is at the specific industry and occupation levels. This may not be something you’ve considered before, but I think that as LMI has become such an integral part of colleges in recent years, it is worth spending a few moments thinking about how this works, and why it is so important.
Let me give you an illustration that you can hopefully relate to.
Imagine that you were the owner of a shop, and you wanted to find out the trends for fruit and vegetable sales, both in terms of total sales and all the way down to individual varieties. In order to achieve your aim, you create four categories, beginning with the umbrella “Fruit & Veg” category at the top, which is then split into two subsections, one for “Fruit” and one for “Veg”, followed by a third category, “Fruit Types” and “Veg Types”, and finally a fourth level – “Varieties” – which goes right down to specific varieties of particular types of fruit and vegetable.
You decide that you want to find out how different varieties of apples have been doing over the past ten weeks, in order to plan more effectively in the future, and so you allocate the task of finding this information out to your deputy manager. Off he goes and quickly works out the total of “Fruit & Veg” sales, as well as the breakdown between “Fruit” and “Veg”. However, when he attempts to find out the more specific details of types and varieties, it becomes apparent to him that this is going to involve an awful lot more time and effort than he spent finding out the previous categories. So he decides to cut some of that work out by taking the sales trend for “Fruit” – the second category of specificity – and applying it to all the individual types of fruit and to all the individual varieties.
When he gives you the results, you are initially pleased to see that sales of Braeburns went up by 3% over the ten week period. However, when you come to the figure for Jazz, Cox, and Granny Smiths, you are surprised to find out that they also apparently grew at 3% as well. This seems highly unlikely to you, and so to test your doubts you go through the same exercise yourself, but this time being far more thorough in your methodology than your deputy.
As you complete the exercise, your suspicions are confirmed. Whereas “Fruit” did indeed grow by 3%, apples themselves only rose by 2% overall, and as for apple varieties, Granny Smith sales increased by 10%, Cox by 7%, Jazz by 1%, and Braeburns actually fell by 10%. Needless to say, you are not exactly best pleased with your deputy, but thankful that you discovered the error before it cost you a lot of money in waste from over ordering and missed opportunity.
What has this got to do with Labour Market Information? Well, LMI actually works on a remarkably similar basis to the story mentioned above. Just as there were four categories in the illustration, so with LMI there are four distinct Standard Industry Classifications (SIC) and four distinct Standard Occupation Classifications (SOC). SIC and SOC 1 are the equivalent of the overarching “Fruit & Veg” category and include general categories of industries and occupations such as Manufacturing and Professional Occupations. Underneath these categories there are increasing levels of specificity, with SIC 2 and SOC 2 being at the “Fruit” level, SIC and SOC 3 at the “Type” level, and finally SIC and SOC 4 being at the most specific “Variety” level.
Just as in the illustration, finding out the data at the first two levels is a fairly simple exercise. However, when you get down to the more specific categories – SIC 3 and 4, and SOC 3 and 4 – things are far more complex because – as mentioned above – there are “holes” in the data. In fact, it is so complex that we needed several years of research, plus the work of a team of expert economists, before we had a methodology that we could confidently go to colleges and claim was accurate and robust!
Why was it so important to go to all that trouble? For the same reason that a shop owner wanting to know the sales of individual varieties needs to go to a lot of trouble: because taking the figures at the 2-digit SIC or SOC level and assuming that the industries or categories beneath them could well see you coming up with the same kinds of wrong answers that the deputy in the shop example came up with.
Let’s take an example. According to the SOC classification system, Veterinary nurses is a 4-digit occupation and it comes under the SOC 2 level Caring Personal Service Occupations. But also under Caring Personal Service Occupations are a variety of disparate occupations including Teaching Assistants, Pest Control Officers, Care escorts and Undertakers. Now just as you would have been suspicious to find out that all varieties of apples grew at the same rate, so you would probably be suspicious if we gave you data suggesting that Veterinary nurses are growing at the same rate as Teaching Assistants, Pest Control Officers, Care escorts and Undertakers. I mean, I know that economies are interconnected, but they’re not that interconnected! In reality, each of these occupations has some quite different economic drivers affecting them.
Just to put a few figures to this, Caring Personal Service Occupations in Lancashire, for instance, grew by 18% over the past five years. However, underneath this “Fruit” level, down in the “Variety” level, Veterinary nurses actually grew by 37% and Teaching Assistants by 16%, whilst Pest Control Officers, Care escorts and Undertakers actually fell by 7%, 14% and 2% respectively.
Do you see the importance of this? If we were to take the same kind of approach to finding out LMI at the 3 and 4 digit levels as the deputy in the shop did to finding out the “Types” and Varieties”, we would end up giving you data that could very well end up leaving you with an impression about an industry or occupation that is the exact opposite of what is really happening. I hardly need to tell you that this could end up being costly for your organisation, if you were to base decision making around it.
As I mentioned at the start of this article, Labour Market Information, as such, is unlikely to get everyone in the FE sector excited, yet its applications and opportunities probably will. But as someone who has been involved for years in helping colleges use LMI to open up these opportunities, and has seen first-hand both the benefits of using data with robust methodology and the drawbacks of using data without such a robust methodology, I have felt for some time that it would be good to try to help the sector better understand some of the complex issues involved. I hope this piece has given you some insight into this, and that it has helped you understand a little better the importance of using the best available data and not just what is to hand, irrespective of its quality.
Andy Durman is VP of UK operations for the labour market information firm Economic Modelling Specialists International
Responses