Are we using the right tools to combat recruitment bias?
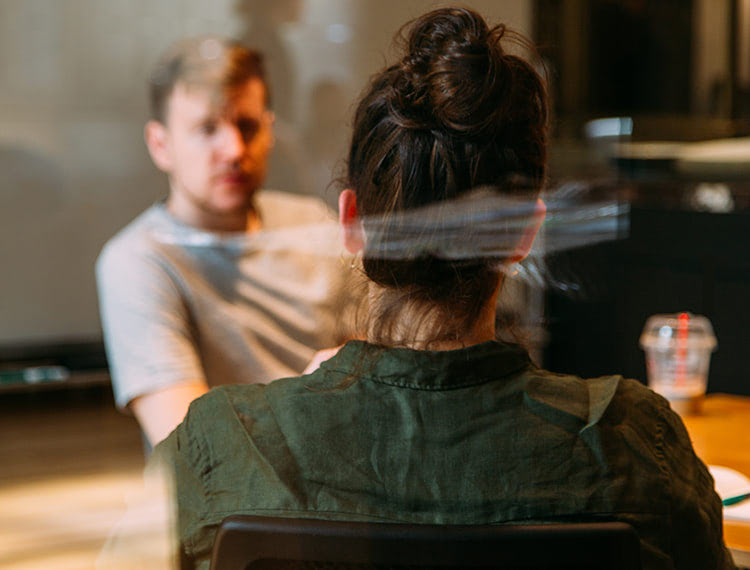
If a CV is on the borderline of what a recruiter is looking for, it’s twice as likely to be shown leniency and accepted if it belongs to a man with a white-sounding name, compared to one belonging to a man with an ethnic-sounding name; even if their qualifications and experience are exactly the same . This may sound like a fact from the last century, but we are still seeing all kinds of prejudice – not just racial – prevailing within the recruitment process.
Although very few of us would like to think that we might judge someone by their race or gender, to some degree, we all make decisions that are influenced by bias. This is because the majority of our decisions are the result of often unconscious thought processes, and recruitment is no different. In fact, this implicit bias has an overwhelming impact on the types of people who are awarded roles.
Whilst there are some firms making great progress in addressing this issue, we still aren’t close to where we should be. One reason for this, is that despite their proactivity, there are firms still implementing solutions that seem to tick all the right boxes in promoting a fair and unbiased process, but instead, are only exacerbating the issue.
A prime example of this are psychometric ability tests such as verbal and numerical reasoning. Whilst both these tests are often used, they are generic, and almost always harm the progression of minority candidates when compared to white candidates. An important reason for this is that these tests measure ‘crystallised intelligence’, which is our knowledge of facts (such as the best use of specific words). Candidates who are first- or second-generation migrants, or from less advantaged socio-economic backgrounds, may have strong potential whilst performing less well on these tests.
Another example are AI programmes. With the rise in online recruiting, they are becoming an increasingly common way to screen applicants, in theory, without the influence of bias because they are objective. However, there are many real-world examples showing how bias affects AI programmes, either through biased or unrepresentative datasets. One example of this was carried out by a civil liberties group using Amazon’s facial software to compare the photos of all federal lawmakers against a database of publicly available mug shots. Astonishingly, the test found the facial software incorrectly matched 28 members of Congress with people who had been arrested; a disproportional number of whom were African-American and Latino members.
In fact, there have been examples of facial recognition programmes that are unable to even identify black individuals as people. Joy Buolamwini, a black computer scientist and digital activist, put this theory to the test by wearing a white mask and found the programmes that previously wouldn’t recognise her, suddenly had no problem.
Automated processes clearly aren’t yet sophisticated enough to decide who should – or shouldn’t – be considered for an open position. In addition, the majority of these tests will ultimately result in a face-to-face interview if a candidate is successful, which simply delays human bias until this point.
Naturally, this situation is not much better at interview stage. In an interview scenario, a recruiter might ask a candidate to describe an experience. The candidate might use a word, such as “challenging” which begins to paint a picture in the recruiter’s mind, but without specific details, there is room for their unconscious mind to fill the gaps with biased assumptions.
Many psychologists believe that the problem lies with ambiguity. Without realising, we are constantly trying to apply reason and logic to the world around us. As exemplified above, a recruiter will read a candidate’s CV and subconsciously build an image of their background and capabilities from their qualifications, their experience; even their name.
One way to counteract this is through situational judgement; i.e. asking candidates questions that will yield objective information, rather than vague or subjective answers. After every question, the level of evidence the candidate has supplied should be analysed and the candidate asked for more detail if needed. This allows our conscious brain to do most of the decision-making, minimising the potential for bias to fill in the gaps.
While psychometric ability tests have been found to significantly reduce the chances of some ethnic minority individuals, situational judgement tests have been found to provide a much more diverse range of candidates. Presenting interviewees with scenarios that they might encounter in their prospective role and asking them to explain how they would respond, is a great way of gaining a subjective impression of a candidate’s mindset. This makes sure that role requirements are clearly assessed, improving selection outcomes. These tests are also capable of ascertaining the future performance of candidates, ultimately saving employers time and money in hiring the correct talent straightaway.
So, whether it be for graduate or board-level appointments, we need to create processes that minimise the risk of human bias in the recruitment process. Simply making recruiters aware of their inherent biases isn’t enough. In order to eradicate bias altogether, there needs to be a conscious effort placed on understanding our decisions and equipping recruiters with the right set of tools.
James Meachin, Managing Psychologist, Pearn Kandola
Responses