AI Barometer in Education
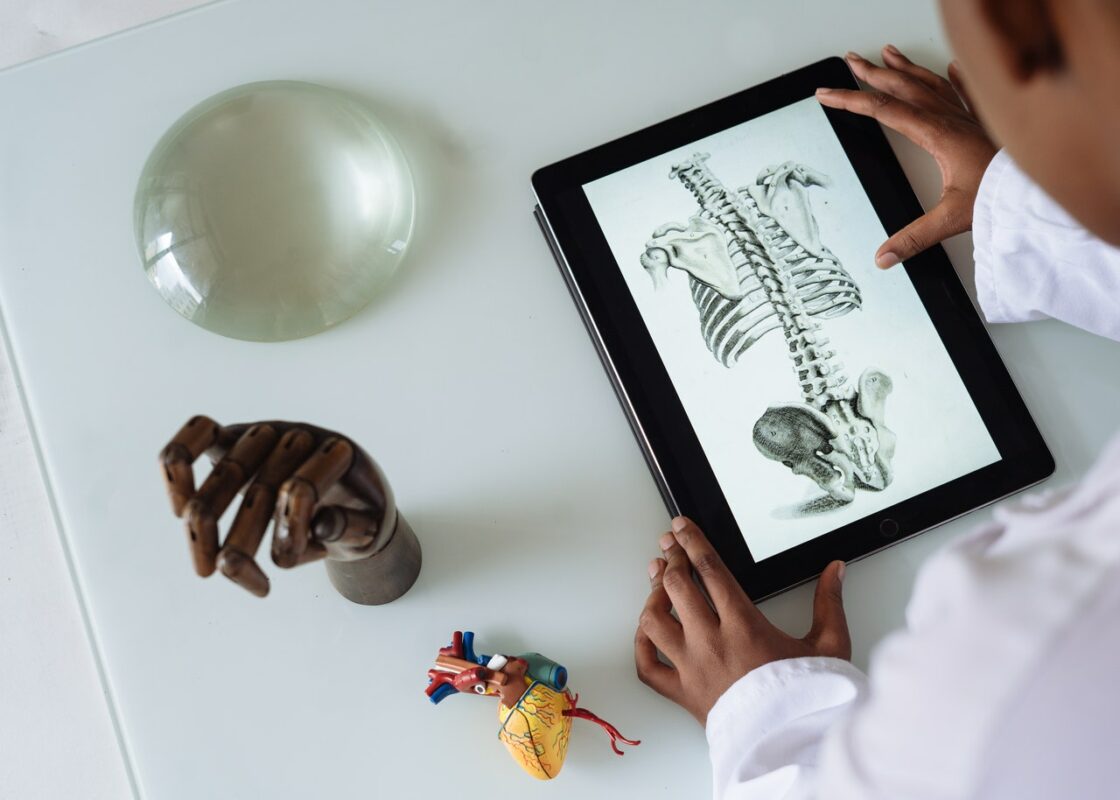
Augmentative technologies could address some of the key challenges facing education systems: Edtech could potentially play a large role in implementing scalable solutions to issues such as the administrative burden on teachers, particularly where applications augment (rather than replace) aspects of the teaching and learning process.
The UK has a considerable edtech sector: The UK is currently home to around 25% of European edtech business, and the OECD predicts that AI systems are likely to become pervasive through the education sector in the medium term, implying potential for the UK to grow and be a leader in this field.
The current market targets only a narrow slice of a complex field: However, education is a complex and diverse field, with the role that data-driven technology can play varying greatly depending on the level of education, subject matter, and preferred pedagogical approaches, to name a few of the most significant factors. Our expert panel highlighted that current market offerings are targeted at relatively narrow subsets of these factors (e.g. school-level STEM-subject learning platforms offering limited pupil feedback), and that many of the most promising opportunities (such as teacher-augmentative personalised learning support) are not currently well developed or catered to.
The edtech market suffers a number of challenges: The market for data-driven edtech products is difficult for both vendors and educators to navigate, due to the diffuse nature of education commissioning. Educational institutions such as schools have little in the way of centralised or standardised processes for edtech procurement, and there are few tools to support them in understanding the benefits of technology use, whether products meet their needs, are appropriate for their learners, or appropriately mitigate risks involved in their use.
Low confidence in technology slows adoption: Concerns around the effectiveness of data-driven technology, its impacts on educator and learner privacy, and the risk of bias are negatively affecting educator trust in data-driven technologies, acting as a barrier to adoption and successful integration into education.
State of Play
Educators face many challenges, including increasing pressure to deliver high quality education to complex student needs, a global shortfall of around 69m teachers, and staff concerns around excessive workload, which is often cited as a primary reason for leaving the teaching profession.
The pandemic saw a sudden and sustained period of remote learning imposed across the education system, and its impact continues to be felt in consequent learner support needs (i.e. ‘catching up’), and continuing disruption to physical learning environments.
The UK’s ‘digital divide’ has presented challenges in delivering education digitally[1], and data shows a significant move towards online for school education delivery. Despite the shift to remote learning and the growth of new tools and technology in education, there is broad consensus that many valuable aspects of face-to-face education cannot be viably substituted for digital alternatives.
While education outcomes are driven by a wide range of factors both within and outside the limits of the education system, data-driven technologies within the education sector (‘edtech’) primarily address three broad applications: ‘teacher-facing’ technologies seek to alleviate administrative and pedagogical pressures on educators; ‘learner-facing’ systems offer opportunities to increase levels of personalisation in learning pathways; and ‘system-facing’ technologies can augment administrators’ and planners’ decision-making capabilities, for example in delivering effective inspection.
Educator-facing systems
These offer numerous solutions to the administrative and pedagogical challenges faced by educators.
- Predictive analytics offer teachers improved understanding of skills gaps, students at-risk of failing assessments or dropping out, which can then be used to augment decisions around mitigations and learning pathways. They can also support adaptive group formation looking at learner knowledge and skill levels, and similarity of interests. More advanced applications use biometric data such as eye tracking and facial expressions to affective states, improved understanding of levels of engagement and educational resource design.
- Aggregated data on students’ attainment and behaviour in educational contexts can be used to inform teachers’ pedagogical approaches, facilitating experimentation in curriculum delivery and classroom management (e.g. automated seating plans and behaviour management tools which monitor pupils’ attendance, interactions and performance, which can inform teachers’ strategies for lesson planning and delivery).
- Natural language processing can support marking written work and provide some forms of feedback, as well as having applications in language learning. These technologies can also facilitate dialogue-based teaching at scale, for example facilitating learning experiences by acting as expert participant or mediator, and monitoring several digital classroom conversations simultaneously.
- Automating routine tasks such as compiling and distributing learning materials, marking homework and monitoring student attainment. These contribute significantly to teachers’ workloads and limit their ability to prioritise students’ individual learning needs.
Learner-facing applications
Perhaps the most significant developments in AI education technologies have occurred in the field of Adaptive Learning Platforms (ALPs) and Intelligent Tutoring Systems (ITSs). These claim to offer a highly personalised learning experience, generating recommendations for learning materials and pathways through predictive analytics and real-time feedback. Through ‘educational data-mining’, these platforms promise tailored learning interventions and data-driven identification of skills and knowledge gaps at scale which may be harder to implement in traditional educational contexts. ALPs and ITSs theoretically open up opportunities in continuous assessment design and building understanding of cognitive processes that affect educational attainment (‘metacognition’), which can be used to improve learner motivation and engagement through dynamic support or alternative pedagogical approaches. ALPs are being trialled in traditional educational settings (e.g. the CENTURY platform in UK schools), and are expected to have the greatest level of impact in regions where access to teachers is low.
System-level applications
System-level applications appear to be among the least mature, but offer improved education outcomes at the institutional level (e.g. school or university) and the system level (district, county, region etc). Ofsted, for example, have used supervised machine learning techniques to identify schools requiring full inspection since 2018 (following a trial with the Behavioural Insights Team in 2017). Further opportunities exist in the form of aggregated learner analytics and performance, which can reveal patterns in educational attainment and best teaching practice. Opportunities at this level are limited by barriers to data sharing between educational institutions and uneven levels of research and development across the curriculum (STEM subjects tend to be easier to embed edtech in than the humanities).
Spotlight: International uses of AI in education
Outside of the UK investment in data-driven edtech tools continues to grow. This has been particularly notable in China. China has been investing heavily in developing AI generally, and more specifically in education, since the Chinese government published its “New Generation Artificial Intelligence Plan” in 2017. A report by the Beijing Normal University and TAL Education found that between 2013 and 2019 14.5 billion yuan were invested in AI Ed tech products. Yi-Ling Liu, writing for Nesta, argues that three factors have created the unique conditions for the current AI education boom in China: a government led, national push to develop these technologies; parents’ willingness to pay for their childrens’ education; and, large quantities of data available for training algorithms.
Many of the applications of these technologies in China are similar to those developing in the UK, particularly those that seek to automate simpler, administrative tasks to free up time for teaching staff. However, China does appear to have a more developed adaptive learning ecosystem than the UK. One of the most notable companies in this space is Squirrel AI, a company that couples digital and physical schooling. Squirrel AI uses a combination of human and AI-driven tutors to create personalised learning plans for each pupil. In 2019, Squirrel operated over 2,000 learning centres and was valued at over $1bn.
China also sees growing use of more cutting edge, and in some cases controversial, technologies. For example, reports point to facial recognition cameras and biometric tools being used to measure things like attention levels and emotional states of pupils. A host of Chinese companies offer products built on emotion recognition technology including: EF Children’s English, Hanwang Education, Haifeng Education, Hikvision, Lenovo, Meezao, New Oriental, Taigusys Computing, Tomorrow Advancing Life (TAL), VIPKID, and, VTRON Group. Use of these technologies has not been without controversy in China, in 2019 the Chinese government said that it planned to “curb and regulate” the use of facial recognition in schools.
Opportunities
- The use of data-driven edtech to address societal challenges such as social mobility and scaling up teaching provision were seen as some of the highest benefit, hardest to achieve opportunities, along with related applications such as the promise of tailored learning pathways, using data-driven platforms to understand what learning approaches work best (‘metacognition’) and reducing administrative burdens for teachers. Many potential benefits identified would ultimately improve the scalability and quality of education, increasing social mobility. Our panel noted that a universal barrier to the benefits of much data-driven edtech was learner access to connected digital devices.
- Generally the most significant opportunities were perceived around applications that augment educators’ capabilities rather than outright replace whole aspects of their role – for example, tools that reduce administrative workload, help teachers find resources, or provide supplemental after-school roles in helping learners access good quality content. However, these were seen as harder to achieve due to the current edtech market, which was perceived as promoting a narrow conceptualisation of teaching, and prioritising easier to develop tools that automate teaching functionality in contexts where learner responses are easier to grade automatically (e.g. STEM subjects).
- Personalised learning pathways were perceived as being enormously valuable as a prospect – and the focus of most AI research in edtech – but were considered to still be very early in their maturity. These applications could potentially improve the quality of education by tailoring teaching to learner needs and informing educators on how effective their methods are, but current market offerings were perceived to primarily provide increased scalability and efficiency through platform-based delivery and generalised feedback rather than necessarily improving the quality of education. At this stage in development, such systems potentially provide the greatest value where particular subjects are otherwise unavailable to learners (e.g. if not offered by a child’s school). The panel noted there were currently few apparent examples of edtech being developed to improve and scale the training of educators themselves.
- Data-driven marking systems hold similar promise in improving feedback for learners while also reducing educator workload – but were also considered to be at an early stage in development. Most AI-based marking systems cannot currently look beyond identification of basic variables that may indicate the quality of an answer and cannot follow written arguments, making them more suited subjects with more easily computable assessment metrics, with STEM subjects receiving a higher degree of developers’ attention than the humanities, and very few applications of edtech tools in subjects that require practical/hands-on supervision. Our panellists also observed that reading students’ work and the assessment cycle generally is one of most important ways that teachers track student ability, progress and needs, so marking systems will need careful integration into assessment processes as they mature in capability. Our panel emphasised that data-driven systems should not solely determine assessment outcomes, but support broader assessment initiatives (e.g. some applications give formative feedback to the student, providing a learning opportunity rather than acting as a marking ‘gateway’). However, they noted that there were significant opportunities around fairness and consistency in marking examinations, for example using AI models to generate indicators of human marking accuracy.
- Our panellists observed that the evidence base for data-driven technology improving education outcomes is not fully developed, making it hard to assess how significant some opportunities will be, particularly as tools will vary in utility by subject, learner groups and education environments. Many prospective applications of data-driven technology in this sector require culture change involving educators, learners, and families to fully realise, and our panel noted that presently there are few clear lines between the accountability frameworks that teachers are held to and outputs of typical edtech applications.
Opportunities quadrant
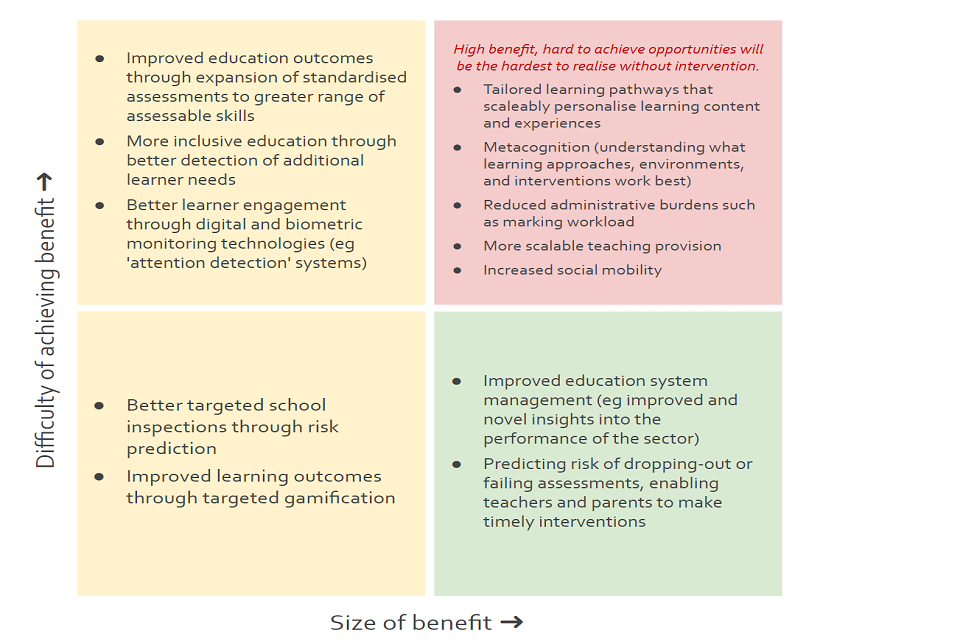
Risks
- Algorithmic bias was broadly seen as the highest likelihood and highest impact risk by our panel, in line with other sectors where data collection and processing is primarily of a personal and sensitive nature, and where decisions supported by data-driven technology have significant impacts on individuals’ choices and opportunities.
- There are a broad range of approaches to teaching, some of which are easier to automate, scale or support using data-driven technology – but intense debate exists over which approaches are most effective and appropriate for different kinds of learners and learning environments. Some panellists were concerned that mismatches between market offerings and educator needs meant that most edtech products were automating the easiest (but not necessarily the most effective) approaches to teaching, with the risk of automating poor pedagogic practices as they are integrated into education environments.
- Our panel also raised a set of privacy and autonomy concerns regarding data collection and retention in education environments, primarily on learners but also on education professionals. This data can often be both personal and sensitive, and is typically very easy to de-anonymise given its specificity.
- Education professionals, learners and their guardians are unlikely to have a clear understanding of how data is used by third-party vendors in many instances. Collection of data by data-driven tools such as learning platforms or in-classroom applications is qualitatively different to earlier edtech in terms of scale, detail, and subsequent inferences drawn. Third party access to such data is typically much easier than under the safeguards of national datasets such as the National Pupil Database, where researchers must request access for each query and specify what data will be used, for what purpose, and how it will create public good. Easier access and use enables faster product iteration, but relies on the provision of data subject consent, which panellists highlighted is not always meaningfully obtained.
- For school-level learners, data consent is typically provided by parents, and requested by schools under general terms and conditions that may not give a clear picture of how young learners’ data may actually be used, making it challenging for data subjects to understand and protect their interests. Our panel highlighted concerns around longer-term data stewardship and the unclear extent of data subject control over the retention or deletion of their data[2], highlighting examples elsewhere in the public sector such as primary care data where individuals are given more nuanced consent and control options.
- Panellists expressed concern about some of the more privacy-invasive applications apparent in international contexts such as ‘attention recognition’ monitoring systems, but saw such applications as raising fewer immediate risks in a UK context due to their low uptake.
- Given the range of challenges facing education systems, panellists highlighted the significant opportunity cost of not maximising the benefits of technology use in education, and the key role of institutional, professional and parental trust in driving adoption of such use, against a historic background of difficult edtech market conditions and controversial use cases in the sector. The absence of specific regulatory frameworks or guidance addressing some of these risks was seen as an aggravating factor.
Risks quadrant
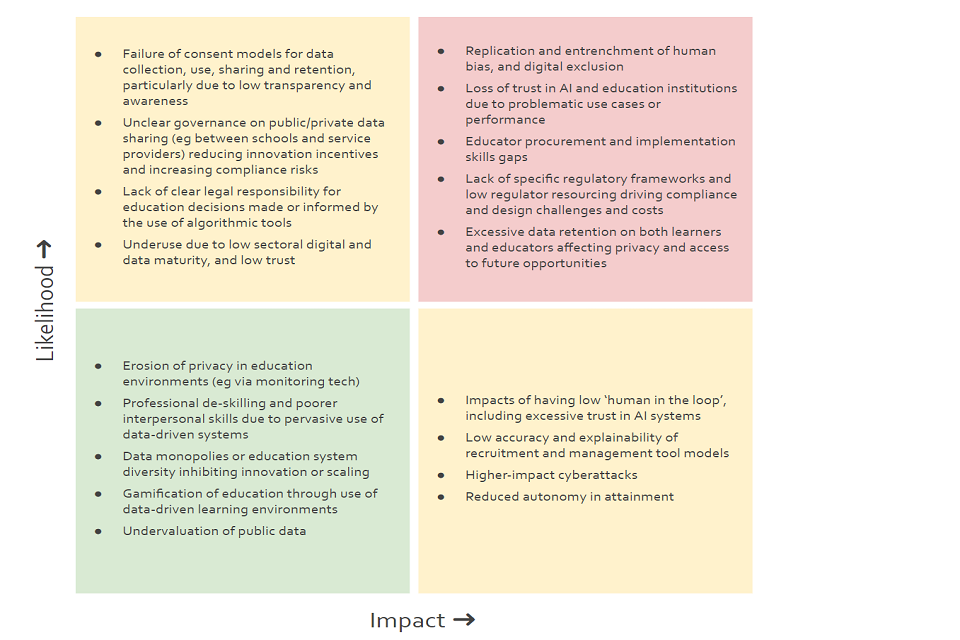
Major risk theme – algorithmic bias
- Predictive analytics and AI models are apparent in many educational applications including assessing learners’ performance (e.g. marking exams) or estimating their attention or engagement levels. The consequences of these decisions on learners’ lives can be very significant, both individually and cumulatively.
- As with other contexts, the data available to train AI systems is unlikely to be free of bias, and can reflect existing social biases both at the learner level (e.g. an AI system being less accurate at interpreting neurodivergent forms of expression due to poor representation in training data) or at system levels (e.g. unfair algorithmic outcomes that result from historic data on education outcomes from different socioeconomic groups). Systems will also typically have imperfect information (e.g. on a learner’s home environment).
- As established in the CDEI review into algorithmic bias, the concept of fairness can have many meanings in the context of data-driven technology, and our panel could point to little evidence of coordination of concepts or standards around fairness in edtech at industry level, or among bodies such as exam boards developing such technology in-house.
- As well as the direct impact of algorithmic bias on learners, difficulties in understanding whether particular products appropriately mitigate it drive mistrust of edtech within the sector (as detailed further below), acting as a barrier to adoption. Our panel emphasised the value of auditing and assurance approaches to mitigating bias and increasing educator confidence in edtech.
Major risk theme – effective procurement and integration
- Our panel highlighted a number of factors that inhibit the uptake of data-driven edtech and drive other risks such as the deployment of biased algorithmic tools. These included a lack of consistent procurement procedures and training for public sector edtech customers, a lack of guidance around edtech data governance, uncertainty as to the appropriate degree of human involvement in decisions regarding learning interventions, and around the levels of performance required by a tool before real-world deployment. Our panel noted some regulators have relatively little capacity to engage with these technology-specific challenges, or ensure product claims are accurate.
- Some peer-led edtech review platforms exist to help educators navigate the marketplace and some evaluative studies can help educators understand which technology-based interventions are effective (e.g. those by the Education Endowment Foundation), but these do not include detail to the level of specific products on the market, and there are no formal edtech assurance schemes. These difficulties can lead to inefficient cyclical purchasing, with educators successively trialing and discarding products that do not meet their needs.
- Beyond procurement skills, our panel indicated there appeared to be relatively little support available to ensure educators have the digital and data skills needed to integrate edtech tools into teaching practices effectively, particularly in online contexts, or as part of teacher training. Our research found little evidence of edtech tools being leveraged to improve the training of educators themselves.
Barriers
Barriers to responsible innovation are the issues that prevent us from maximising the benefits of data-driven technologies, and which prevent harms from being appropriately mitigated.
Limited market understanding of educator needs
- Our panel identified the misalignment between the solutions being provided by the edtech market and educator/learner needs as one of the most significant barriers to responsible innovation in this sector, among a range of market-based challenges. A substantial proportion of the edtech market was perceived to be targeting the ‘low hanging fruit’ of potential applications, and focused around replacing aspects of teaching, rather than augmenting and scaling the capabilities of educators. Relatively few educators are involved in the development of edtech tools or teaching content, resulting in a limited market understanding of areas where such technology could have the best impact.
Poorly accessible market information
- The market is challenging for both vendors and educators to navigate. As highlighted in the major risk theme above, edtech customers struggle to investigate and understand differences between products at a technical level, limiting their ability to procure the most appropriate tools for their needs. Research and development costs are high due to the nature of showing improved education outcomes, and there are relatively few financial and structural incentives for vendors to develop more specialised support offerings; the decentralised management of schools means educators tend to purchase on a smaller scale (e.g. compared to the commissioning of health services and products), making economies of scale harder for vendors to achieve. The relative immaturity of the market, and lower levels of digital/data maturity across much of the educator sector further disincentivise investment and widespread adoption of edtech.
Low trust in edtech
- Our panel noted the barriers above, along with concerns around privacy risks as factors negatively affecting educator trust in data-driven technologies, as part of broader trust in edtech and concerns around the use of emerging technologies on children – and that this in turn was acting as a barrier to adoption and successful integration into education. Specific concerns included the unclear pedagogical value of some tools and whether they might reinforce bad practice, long term data retention, impact on learners’ social skills, and issues around controversial use cases of algorithms in assessments and privacy-invasive applications deployed internationally, including examples of where examination bodies had had to place edtech development on hold due to public concerns about the impact on learners. Panellists cited involving educators, learners, and learner guardians earlier in development processes as important for addressing many of these issues, and the need for more nuanced consent options around data collection and retention.
Appropriate and available data
- As well as typical data sharing and quality challenges (e.g. a lack of strong data sharing mechanisms between educational institutions, assessment bodies, regulators and industry), education systems also face policy tradeoffs in improving access to relevant data. Education outcomes depend on a broad range of factors, including many outside the educational setting, that it would be impractical and inappropriate to gather data about (e.g. a learner’s home life) and so will not be available to inform predictive analytics and similar technologies.
- A 2019 ONS study found that 60,000 11-18 y/os do not have access to the internet at home, and 700,000 do not have access to a computer, laptop or tablet to access online learning ↩
- For a fuller appraisal of these issues, see for example The Ethics of Using Digital Trace Data in Education: A Thematic Review of the Research Landscape, 2021 ↩
AI Barometer
The CDEI has published the second edition of its AI Barometer, alongside the results of a major survey of UK businesses.From:Centre for Data Ethics and InnovationPublished17 December 2021
Documents
AI Barometer Part 1 – Summary of findings
HTML
AI Barometer Part 2 – Business Innovation Survey 2021 summary
HTML
AI Barometer Part 3 – Recruitment and workforce management
HTML
AI Barometer Part 4 – Transport and logistics
HTML
AI Barometer Part 5 – Education
HTML
Business Innovation Survey 2021
PDF, 604 KB, 43 pages
Details
The CDEI’s AI Barometer is a major analysis of the most pressing opportunities, risks and governance challenges associated with AI and data use in the UK. This edition builds on the first AI Barometer and looks at three further sectors – including recruitment and workforce management, education, and transport and logistics – that have been severely impacted by the COVID-19 pandemic. Alongside the AI Barometer, the CDEI has published the findings from a major survey of UK businesses across eight sectors, which it commissioned Ipsos MORI to conduct to find out about businesses’ readiness to adapt to an increasingly data-driven world. The survey was undertaken between March and May 2021.
To produce the AI Barometer, the CDEI conducted an extensive review of policy and academic literature, and convened over 80 expert panellists from industry, civil society, academia and government. It used a novel comparative survey to enable panellists to meaningfully assess a large number of technological impacts; the results of which informed a series of workshops.
Key findings
- The CDEI’s analysis highlights the ‘prize to be won’ in each sector if data and AI are leveraged effectively. It points to the potential for data and AI to be harnessed to increase energy efficiency and drive down carbon emissions; improve fairness in recruitment and management contexts; and enable scalable high quality, personalised education, in turn improving social mobility.
- In order to realise these opportunities, however, it points to major, cross-sectoral barriers to trustworthy innovation that need to be addressed, ranging from a lack of clarity about governance in specific sectors, to public unease about data and AI are used.
- The report also ranks a range of risks associated with AI and data use. Top risks common to all sectors examined include: bias in algorithmic decision-making; low accuracy of data-driven tools; the failure of consent mechanisms to give people meaningful control over their data; as well as a lack of transparency around how AI and data is used.
- The major survey of UK businesses generated a range of insights. For example, it revealed that businesses that have extensively deployed data-driven technologies in their processes would like further legal guidance on data collection, use and sharing (78%), as well as subsidised or free legal support on how to interpret regulation (87%).
Next steps
The CDEI’s work programme is helping to tackle barriers to innovation. It worked with the Recruitment and Employment Confederation to develop industry-led guidance to enable the responsible use of AI in recruitment. It has also set out the steps required to build a world-leading ecosystem of products and services that will drive AI adoption by verifying that data-driven systems are trustworthy, effective and compliant with regulation.
Separately, it is working with the Cabinet Office’s Central Digital and Data Office to pilot one of the world’s first national algorithmic transparency standards, as well as with the Office for Artificial Intelligence as it develops the forthcoming White Paper on the governance and regulation of AI.
Responses